The Real Problem We Should Care About: Bias and Fairness in AI for Healthcare
Introduction
Check our post about AI in Healthcare: Entering a New Era
As Artificial Intelligence (AI) becomes increasingly integrated into healthcare, it promises to revolutionize diagnostics, treatment planning, patient care, and operational efficiency. However, one of the most pressing issues that arise with the deployment of AI in healthcare is the problem of bias and fairness. This blog post delves into the critical concerns surrounding bias in AI healthcare systems, the implications for patient care, and the steps necessary to mitigate these challenges to ensure equitable and effective healthcare for all.
Understanding Bias in AI
Bias in AI refers to the presence of systematic and unfair discrimination in the outcomes produced by AI systems. This can occur at various stages of AI development and deployment, including data collection, algorithm design, and implementation. Bias can stem from several sources:
- Historical Bias: AI systems trained on historical data can inherit existing biases present in that data. For example, if historical medical data predominantly represents certain demographics, the AI system may perform poorly for underrepresented groups.
- Sampling Bias: If the training data is not representative of the entire population, the AI model may produce biased outcomes. This occurs when certain groups are overrepresented or underrepresented in the dataset.
- Measurement Bias: This occurs when the data used to train AI systems is inaccurately measured or recorded. For instance, if certain health conditions are underdiagnosed in specific populations, the AI system might not learn to recognize these conditions effectively.
- Algorithmic Bias: The design and parameters of the AI algorithm itself can introduce bias. Choices made by developers regarding which features to include, how to weigh them, and how to handle missing data can all contribute to biased outcomes.
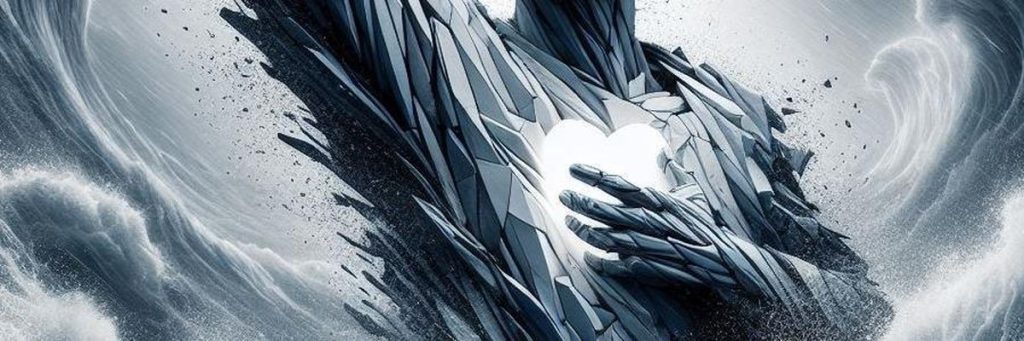
The Impact of Bias in AI Healthcare Systems
- Disparities in Diagnostics and Treatment: Biased AI systems can lead to disparities in diagnostic accuracy and treatment recommendations. For example, an AI model trained predominantly on data from one racial or ethnic group might underperform when applied to patients from other groups, resulting in misdiagnoses or inappropriate treatments.
- Inequitable Access to Care: AI-driven healthcare tools might not be equally accessible or effective for all populations. For instance, telemedicine platforms utilizing AI might have higher accuracy and better user interfaces tailored to certain demographics, leaving others with suboptimal care.
- Erosion of Trust: If patients and healthcare providers perceive AI systems as biased or unfair, it can erode trust in these technologies. This can hinder the adoption of potentially beneficial AI tools and negatively impact patient outcomes.
- Legal and Ethical Implications: Biased AI systems can lead to legal and ethical issues, including violations of anti-discrimination laws and ethical standards. Healthcare providers could face legal repercussions if AI-driven decisions disproportionately harm certain patient groups.
Addressing Bias and Ensuring Fairness
- Diverse and Representative Data: Ensuring that training datasets are diverse and representative of the entire population is crucial. This involves collecting data from a wide range of demographic groups, including different ages, genders, races, ethnicities, and socioeconomic backgrounds.
- Bias Detection and Mitigation: Implementing methods to detect and mitigate bias during the AI development process is essential. Techniques such as fairness-aware machine learning algorithms, re-sampling, re-weighting, and adversarial debiasing can help reduce bias in AI models.
- Transparent and Explainable AI: Developing transparent and explainable AI systems can help identify and address biases. Explainable AI provides insights into how decisions are made, allowing developers and users to understand the reasoning behind AI outputs and identify potential biases.
- Inclusive Design and Development: Involving a diverse group of stakeholders in the design and development of AI systems can help ensure that multiple perspectives are considered. This includes healthcare professionals, ethicists, patients, and representatives from underrepresented groups.
- Regulatory Oversight and Standards: Establishing regulatory frameworks and standards for AI in healthcare can ensure that AI systems are developed and deployed ethically and responsibly. Regulatory bodies can provide guidelines for data collection, algorithm development, and bias mitigation.
- Continuous Monitoring and Evaluation: AI systems should be continuously monitored and evaluated for bias and fairness throughout their lifecycle. This involves regularly updating models with new data, retraining algorithms, and assessing the impact of AI-driven decisions on different patient groups.
- Education and Training: Educating healthcare providers, AI developers, and policymakers about the importance of bias and fairness in AI is essential. Training programs can raise awareness about potential biases and provide tools for addressing them.
Real-World Examples and Case Studies
- Algorithmic Bias in Medical Imaging: Studies have shown that AI models for medical imaging, such as those used to detect skin cancer, may perform better on images of lighter skin compared to darker skin. This highlights the need for diverse training datasets and fairness-aware algorithms.
- Disparities in Cardiovascular Risk Prediction: AI models predicting cardiovascular risk have been found to be less accurate for women and minority groups compared to men and majority groups. This can lead to underestimation of risk and inadequate preventive measures for these populations.
- Bias in Electronic Health Records (EHR) Systems: AI tools that analyze EHR data can inherit biases present in the records. For example, if certain conditions are underdiagnosed in specific populations, the AI system might not learn to recognize these conditions effectively, leading to biased outcomes.
Conclusion
Bias and fairness in AI for healthcare is a critical issue that must be addressed to ensure equitable and effective patient care. As AI continues to transform healthcare, it is essential to recognize and mitigate biases in AI systems to prevent disparities in diagnostics, treatment, and access to care. By adopting diverse and representative data practices, implementing bias detection and mitigation techniques, and fostering transparency and inclusivity in AI development, we can harness the full potential of AI while ensuring fairness and equity for all patients. The journey towards unbiased and fair AI in healthcare is ongoing, but with concerted efforts from all stakeholders, we can create a more just and effective healthcare system.
Want to hear more, explore our blog post about The Importance and Impact of Digital Health
One thought on “Bias and Fairness in AI for Healthcare”
Hey people!!!!!
Good mood and good luck to everyone!!!!!