Generative Artificial Intelligence: A Comprehensive Exploration
Introduction
Artificial Intelligence (AI) has been transforming various sectors of our society, from healthcare to entertainment, but one of its most groundbreaking advancements is in the realm of generative AI. Unlike traditional AI systems that operate based on pre-defined rules and data inputs, generative AI creates new content, such as text, images, music, and even complex simulations. This blog post delves deeply into the world of generative AI, exploring its development, underlying technologies, applications, ethical considerations, and future prospects.
Understanding Generative AI
What is Generative AI? Generative AI refers to a category of artificial intelligence systems that can generate new content or data that is similar to the input data it has been trained on. This capability is achieved through models that can understand and mimic patterns in the data, allowing them to produce novel outputs.
Key Technologies Behind Generative AI:
- Generative Adversarial Networks (GANs):
- Overview: Introduced by Ian Goodfellow and his colleagues in 2014, GANs consist of two neural networks: the generator and the discriminator. The generator creates new data instances, while the discriminator evaluates them for authenticity.
- Function: These two networks are trained together in a process where the generator aims to create data that is indistinguishable from real data, and the discriminator learns to differentiate between real and generated data. This adversarial process continues until the generator produces high-quality data.
- Variational Autoencoders (VAEs):
- Overview: VAEs are a type of autoencoder that learns the underlying distribution of the input data. They encode input data into a compressed latent space and then decode it back to generate new data samples.
- Function: VAEs are particularly useful for generating new data that is similar to the training data, and they are often used in applications requiring smooth interpolation between data points.
- Transformer Models:
- Overview: Transformer models, such as GPT-3 (Generative Pre-trained Transformer 3) developed by OpenAI, use attention mechanisms to process and generate sequences of text. These models have significantly advanced the capabilities of natural language processing.
- Function: Transformers can generate coherent and contextually relevant text by leveraging large amounts of training data and understanding the relationships between words in a sequence.
Applications of Generative AI
- Text Generation:
- Chatbots and Virtual Assistants: Generative AI powers sophisticated chatbots and virtual assistants that can engage in natural and meaningful conversations with users. Examples include OpenAI’s GPT-3 and conversational agents like Google Assistant and Amazon’s Alexa.
- Content Creation: AI can generate articles, stories, poetry, and even academic papers. Tools like AI Dungeon use generative AI to create interactive storytelling experiences, while automated journalism platforms produce news reports based on data inputs.
- Image and Video Generation:
- Art and Design: Artists and designers use generative AI to create original artwork, graphics, and designs. GANs have been employed to produce realistic images, enhance photo resolution, and even create deepfake videos.
- Entertainment: The film and gaming industries leverage generative AI to create lifelike characters, scenes, and special effects. For example, AI can generate realistic human faces, landscapes, and even entire virtual worlds.
- Music and Audio Generation:
- Music Composition: AI composers can create original music tracks in various genres. Tools like OpenAI’s MuseNet and Google’s Magenta use neural networks to generate music that sounds remarkably human-made.
- Sound Design: Generative AI is used to create sound effects for movies, games, and virtual reality experiences, offering a vast range of audio textures and atmospheres.
- Scientific Research and Simulations:
- Drug Discovery: Generative models can design new molecules with potential therapeutic properties, accelerating the drug discovery process. AI-driven platforms like Insilico Medicine use generative techniques to propose novel compounds for medical research.
- Climate Modeling: AI can generate simulations to predict climate change impacts, helping scientists to develop more accurate models and strategies for mitigating environmental challenges.
- Personalized Content:
- Marketing and Advertising: Generative AI can create personalized advertisements, product recommendations, and marketing content tailored to individual preferences. This level of personalization enhances customer engagement and improves conversion rates.
- Education: AI-generated content can provide personalized learning experiences, adapting educational materials to suit the needs and learning styles of individual students.
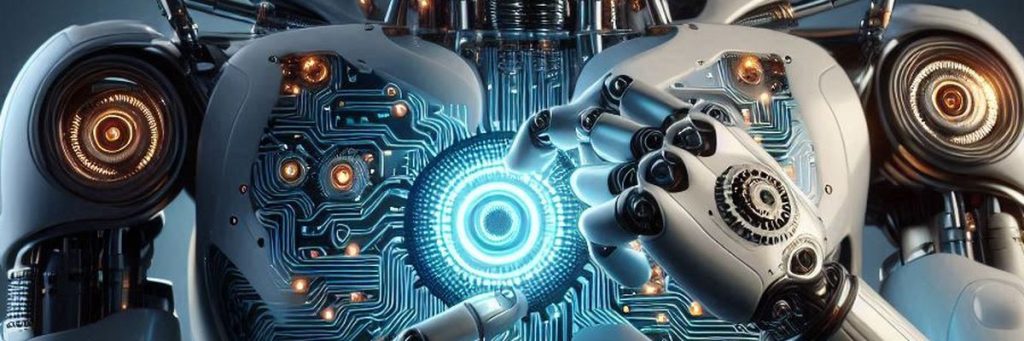
Ethical Considerations and Challenges
- Deepfakes and Misinformation:
- Issue: Generative AI’s ability to create highly realistic images and videos has raised concerns about deepfakes, which can be used to spread misinformation, manipulate public opinion, and harm reputations.
- Mitigation: Researchers and policymakers are developing tools and regulations to detect and combat deepfakes, ensuring that generative AI is used responsibly.
- Bias and Fairness:
Check our detailed post about Bias and Fairness in AI for Healthcare
- Issue: Generative AI models can inherit biases present in their training data, leading to the creation of biased or discriminatory content.
- Mitigation: Efforts are being made to develop fairer AI systems by using diverse training datasets, implementing bias detection mechanisms, and promoting transparency in AI development.
- Intellectual Property:
- Issue: The generation of new content by AI raises questions about intellectual property rights and the ownership of AI-generated works.
- Mitigation: Legal frameworks are evolving to address these issues, with discussions focusing on how to attribute and protect the rights of both creators and AI developers.
- Privacy Concerns:
- Issue: Generative AI can be used to create personalized content based on user data, raising concerns about privacy and data security.
- Mitigation: Stricter data protection regulations and ethical guidelines are necessary to ensure that generative AI respects user privacy and complies with data protection laws.
Future Prospects of Generative AI
- Advancements in Model Architecture:
- Development: Ongoing research aims to improve the architectures of generative models, making them more efficient, accurate, and capable of handling complex tasks. Innovations such as transformer models have already demonstrated significant progress in this area.
- Enhanced Creativity and Collaboration:
- Potential: Generative AI can serve as a creative collaborator, augmenting human creativity in fields such as art, music, literature, and design. This collaboration can lead to the exploration of new artistic styles and the development of novel creative processes.
- Integration with Other Technologies:
- Synergy: Combining generative AI with other emerging technologies like augmented reality (AR), virtual reality (VR), and the Internet of Things (IoT) can lead to innovative applications and immersive experiences. For example, AI-generated content can enhance virtual environments in AR and VR.
- Democratization of Innovation:
- Access: As generative AI tools become more accessible and user-friendly, individuals and small businesses can leverage these technologies to innovate and compete with larger organizations. This democratization of innovation can foster a more inclusive and dynamic economy.
- Ethical AI Development:
- Focus: The future of generative AI will be shaped by ethical considerations, with a focus on developing AI systems that are transparent, fair, and accountable. Researchers and developers are increasingly prioritizing ethical guidelines to ensure the responsible use of generative AI.
- Transformative Impact on Industries:
- Revolution: Generative AI has the potential to revolutionize various industries by automating creative processes, enhancing productivity, and enabling the development of new products and services. Industries such as entertainment, healthcare, finance, and education will continue to be profoundly impacted by generative AI advancements.
Conclusion
Generative AI represents a paradigm shift in artificial intelligence, with the potential to transform how we create, innovate, and interact with technology. From generating text, images, and music to driving scientific research and personalized content, the applications of generative AI are vast and diverse. However, the rise of generative AI also brings forth ethical challenges and societal implications that must be addressed through responsible development, regulation, and public engagement.
As we move forward, the key to harnessing the full potential of generative AI lies in balancing innovation with ethical considerations, fostering collaboration between humans and machines, and ensuring that the benefits of AI are widely distributed across society. By embracing these principles, we can unlock new opportunities for creativity, productivity, and progress, shaping a future where generative AI enhances our lives in meaningful and responsible ways.
2 thoughts on “The Rise of Generative Artificial Intelligence”
AI is important for all kinds of industries at the moment. But it also consumes a lot of energy for every action. It has to be used where it will be effective the most.
Dear Cuan
that is so true, we all look for effective solutions and AI might be key for this.